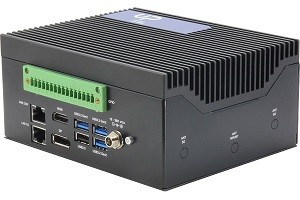
Having covered the first of the teams to reach the finals of the Intel DevCup in part 1 of this two-part Intel® DevCup special, this article will provide insight into the application developed by HackForHealth, a team formed by Rongguang Technology and Kaohsiung Chang Gung Memorial Hospital.
HackForHealth was the second group to make use of the UP Xtreme i12 Edge in the DevCup, reaching the finals. HackForHealth’s application objective was both admirable and ambitious, attempting to use AI to make abdominal aortic aneurysm (AAA) screening more accessible in rural communities that lack experienced medical personnel. The implication of this would be that with the assistance of AI detection, preliminary AAA screening would be made more accurate and accessible to rural communities. Ultimately, the achievement of this goal would result in a greater number of at-risk patients receiving early detection and intervention for a potentially fatal medical condition.